A New Deep Learning Framework for Tuberculosis Growth Detection in 96-Well Broth Microdilution Plates for Antimicrobial Susceptibility Testing
Tuberculosis (TB) is the deadliest infectious disease, with multidrug-resistant TB (MDR-TB) remaining a global issue due to the time-consuming nature of current drug susceptibility testing (DST) methods. While microtitre plates enable high-throughput phenotypic testing, they are difficult to read. The Automated Mycobacterial Growth Detection Algorithm (AMyGDA) is an automated step to interpret those plates but can be inaccurate with low-quality images. To address these challenges, we propose a new framework, namely TMAS (TB Microbial Analysis System), which leverages state-of-the-art deep learning models to detect M. tuberculosis growths in 96-well microtiter plates. TMAS is designed to determine Minimum Inhibitory Concentrations (MICs) quickly and accurately, while simultaneously addressing the issues caused by typical artefacts in plate readings through AI-driven growth detection. Using 4018 images from the CRyPTIC* dataset to train models and develop the framework, TMAS achieved 98.84% essential agreement, outperforming 90.6% of AMyGDA and surpassing the International Organization for Standardization's threshold of ≥ 90%. TMAS offers a reliable automated secondary evaluation to support expert interpretation, improving accuracy and efficiency in TB DST. By advancing MDR-TB treatment, TMAS has the potential to impact global efforts to combat this deadly disease significantly.
*Comprehensive Resistance Prediction for Tuberculosis: an International Consortium
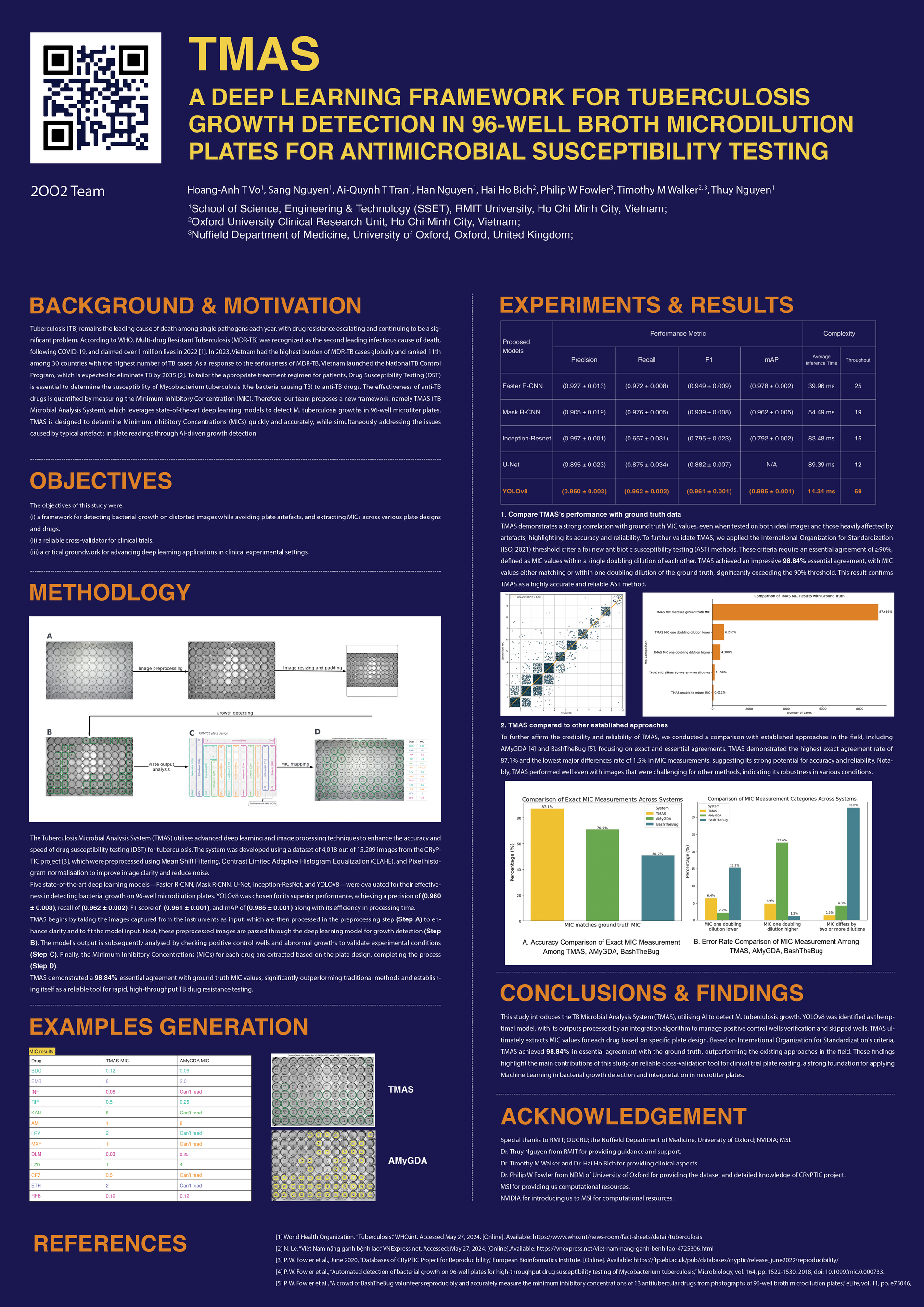