Emotional Flip Reasoning via Stacked Instruction Finetuning of LLMs
Emotions are an integral part of human communication. Emotions extend beyond the confines of internal psychological states, functioning as a mechanism for expressing our subjective experiences and stimulating corresponding responses from others, thereby significantly shaping the dynamics of our communicative interactions. Understanding the triggers for one’s emotional dynamics during their communication with others provides explainability into the underlying drivers of their behaviours and decision-making.
Large language models (LLMs) are increasingly capable of understanding complex aspects of human language, including emotions. Our work presents a novel approach for fine-tuning LLMs to better understand the dynamics of emotion in conversations. Specifically, we focus on the challenge of Emotion Flip Reasoning, which involves identifying the utterances that cause a speaker to shift from one emotion to another. Our framework uses a stacked instruction-based approach, where LLMs are fine-tuned on a series of increasingly complex instructions related to emotions and conversational flows. This allows the model to learn subtle cues and patterns in language that indicate emotional shifts. Our research highlights the potential of LLMs to not only recognise emotions in text but also to reason about the causes and consequences of emotional change in conversational exchanges.
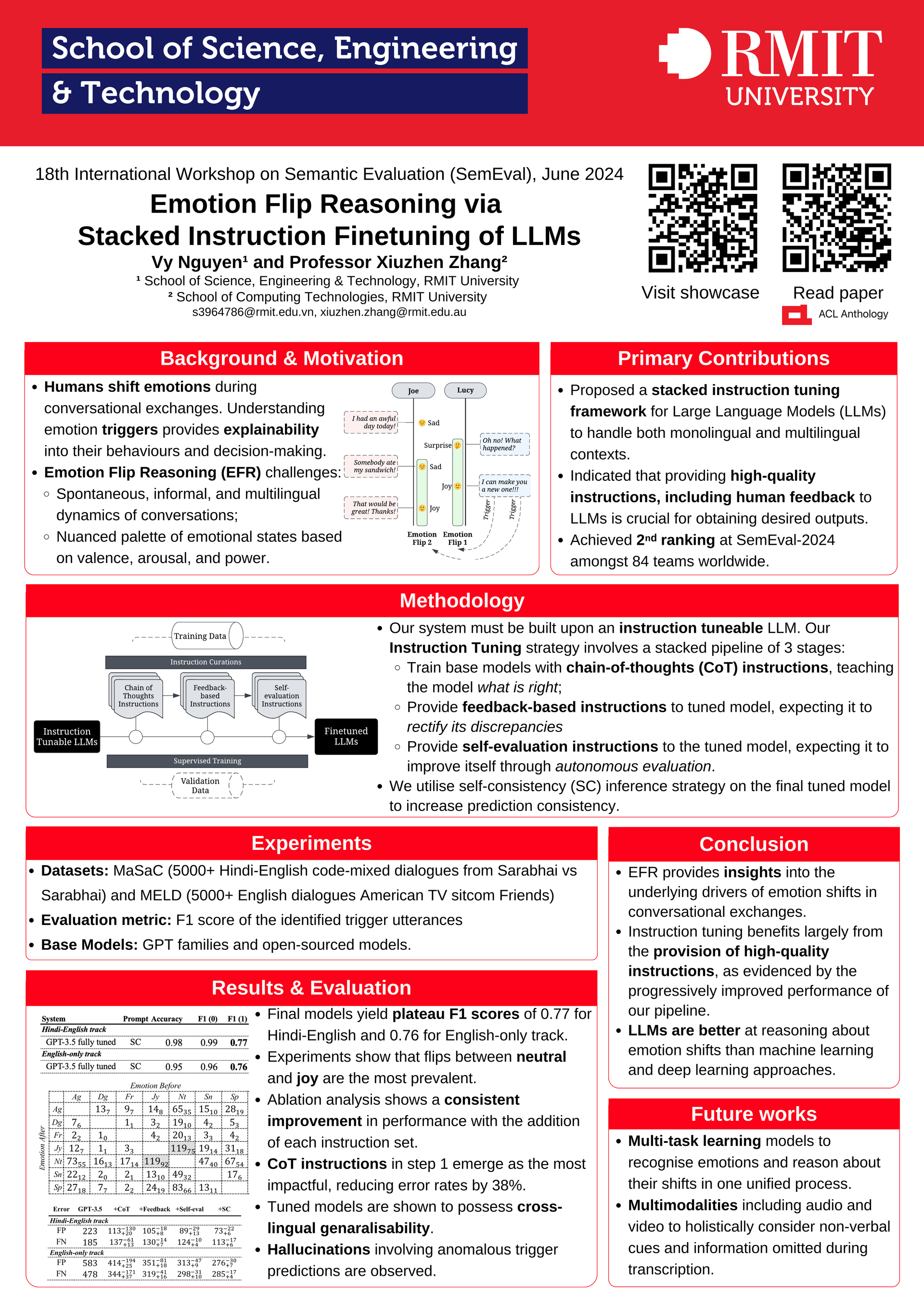