INSECT DETECTION & QUANTIFICATION SYSTEM
Our AI system addresses the critical challenge of pest identification in agriculture. We curate a diverse dataset comprising high-quality images of five common insect species: planthopper, leaf roller, mosquito, thrip, and stem borer. Rigorous preprocessing ensures consistent labeling and optimal model training.
For insect classification, we utilize two robust convolutional neural networks: VGG16 and ResNet50. These models learn discriminative features from the dataset, enabling precise species identification. Additionally, YOLOv5, our object detection framework, identifies insect candidates within images. Bounding boxes drawn around these candidates define regions of interest.
In our integrated workflow, when YOLOv5 detects an insect candidate, we extract the corresponding bounding box coordinates. These ROIs undergo classification using both VGG16 and ResNet50. The combined predictions yield accurate insect species labels.
Our system empowers farmers by providing timely information. Armed with early pest detection, farmers can take proactive measures to protect their crops, minimize damage, enhance yield, and promote sustainable agriculture.
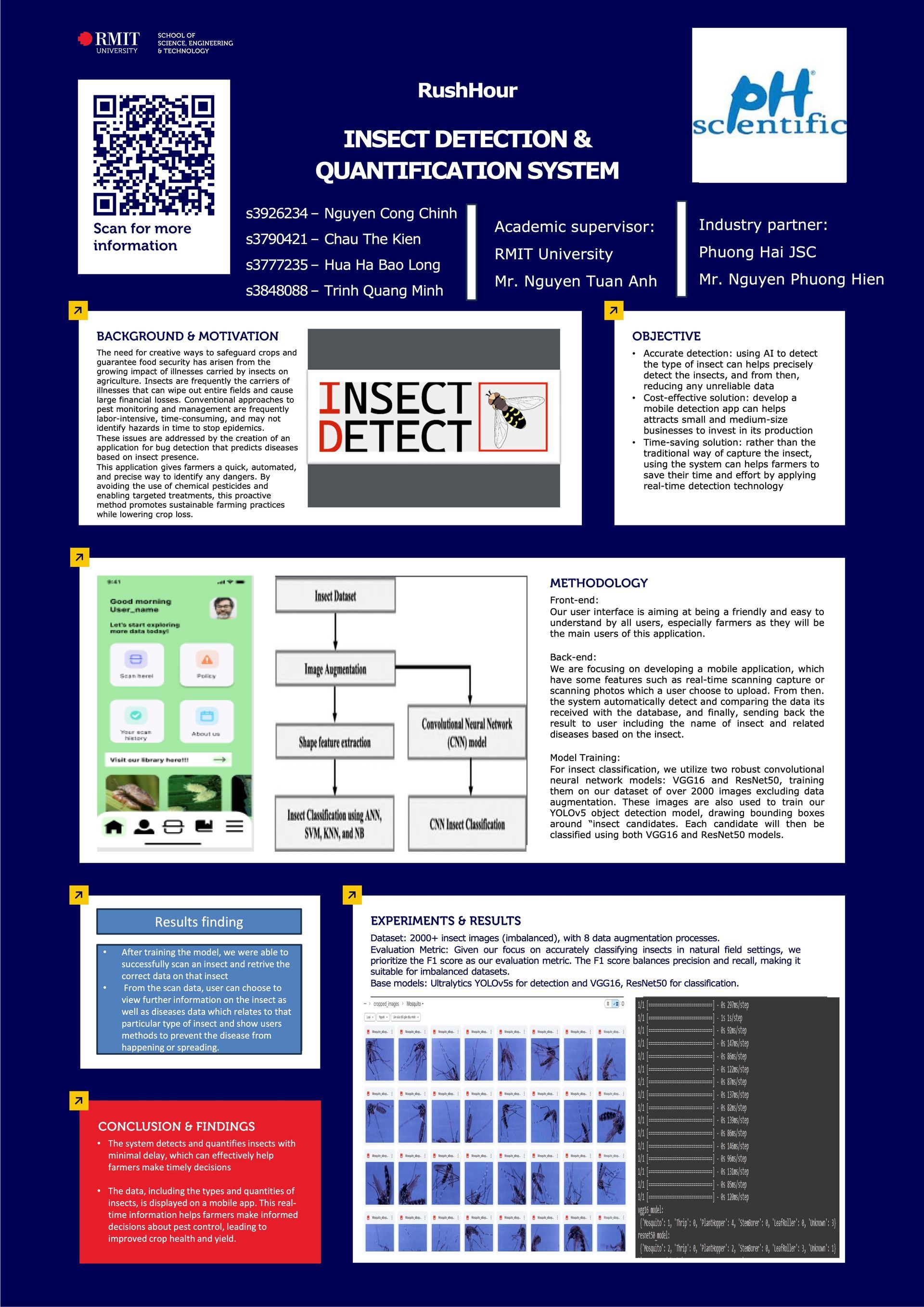